[#8] The Power Law in Venture Capital
An attempt to understand how VC returns exhibit the Power Law, and what it could mean for portfolio construction (Marginal Futility #8)
The normal distribution describes a lot of phenomena we observe in day to day life. Several phenomena depict a central tendency, with majority of observations concentrated tightly around the average and observations that are not close to average are not too far away from it as well. As we move away from the mean, the normal curve would drop off sharply, with close to 95% observations lying within two standard deviations from the mean. A single observation or a small set of observations do not have an out-sized impact on the overall distribution.
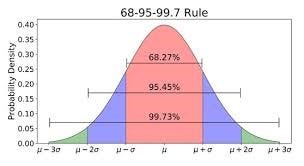
A different, and very interesting, type of distribution is the Power Law. A small number of inputs account for a large percentage of outputs. Power laws have “fat tails” compared to the area under a normal distribution curve which falls off much faster as you move farther along the x-axis. To put it crudely, think of the 80-20 principle as a distribution: 80% of the outcome determined by 20% of the observations.
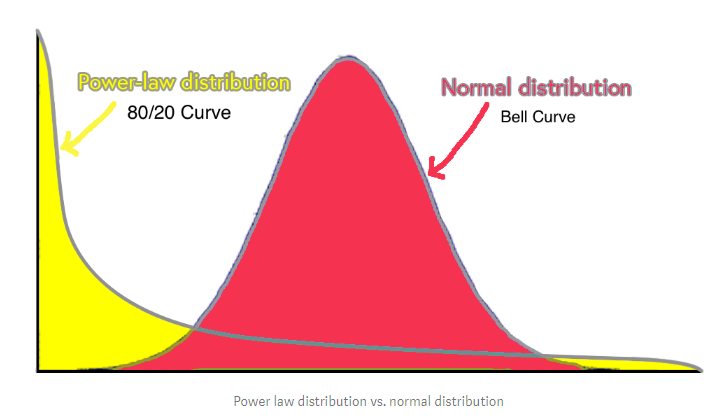
…
A look at VC investment returns
Marc Andreessen of Andreessen Horowitz, one of the largest and most successful VC firms globally, points out:
Each year, of the 4,000 technology startups seeking VC funding, only 200 (or 5%) are seriously fundable, with “15 of those generating 95% of all economic returns…even the top VCs write off half their deals.
We have often heard that several startups (even funded ones) do not do very well. As we will see later, historical data confirms that most VC investments do not do well (often do not lead to any return to the VC), some do reasonably well (say a 2x or 3x return) and a small handful do extremely well (say a 10x or a 25x return or even more). VCs invest around the idea of the last category, they know many (or most) investments won’t do very well, but hopefully a few of them would turn out to be home runs, potentially returning several times the invested amount.
Let’s test this idea by looking at actual venture capital return data. However, accurate venture capital returns data is quite tough to find considering the investee companies are private and deal terms are often not disclosed. Hence, we will consider multiple data sources.
First, a report by Cambridge Associates released in November 2015 which analyzed venture investments from 1995 to 2012, an 18-year period. For the period covered by this analysis, the top 100 investments each year (measured in terms of returns generated) accounted for a percentage of total value creation that ranged from a minimum of 72% of total industry-wide returns in 2012 to a maximum of around 100% of total returns generated across several years. Its interesting to note that on an average, there were more than 4,000 VC investments being made every year.
Essentially, less than 2.5% of the investments were responsible for a bulk of the returns generated by the entire venture capital industry!!
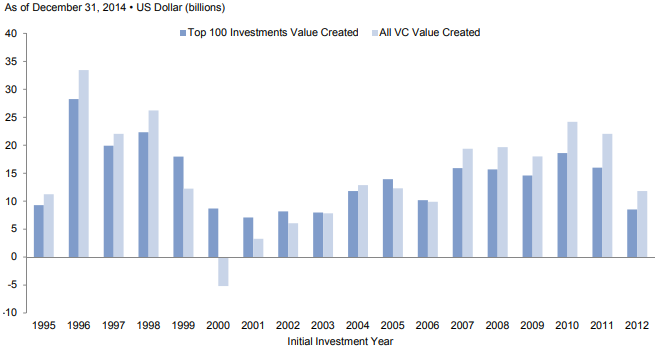
Second, let’s consider the data put out by Andreessen Horowitz. They cite data provided by Horsley Bridge (a large fund-of-funds which invests in several venture funds). As per the data spanning atleast two decades, Horsely’s returns have been highly concentrated. ~6% of investments representing 4.5% of dollars invested generated ~60% of the total returns.
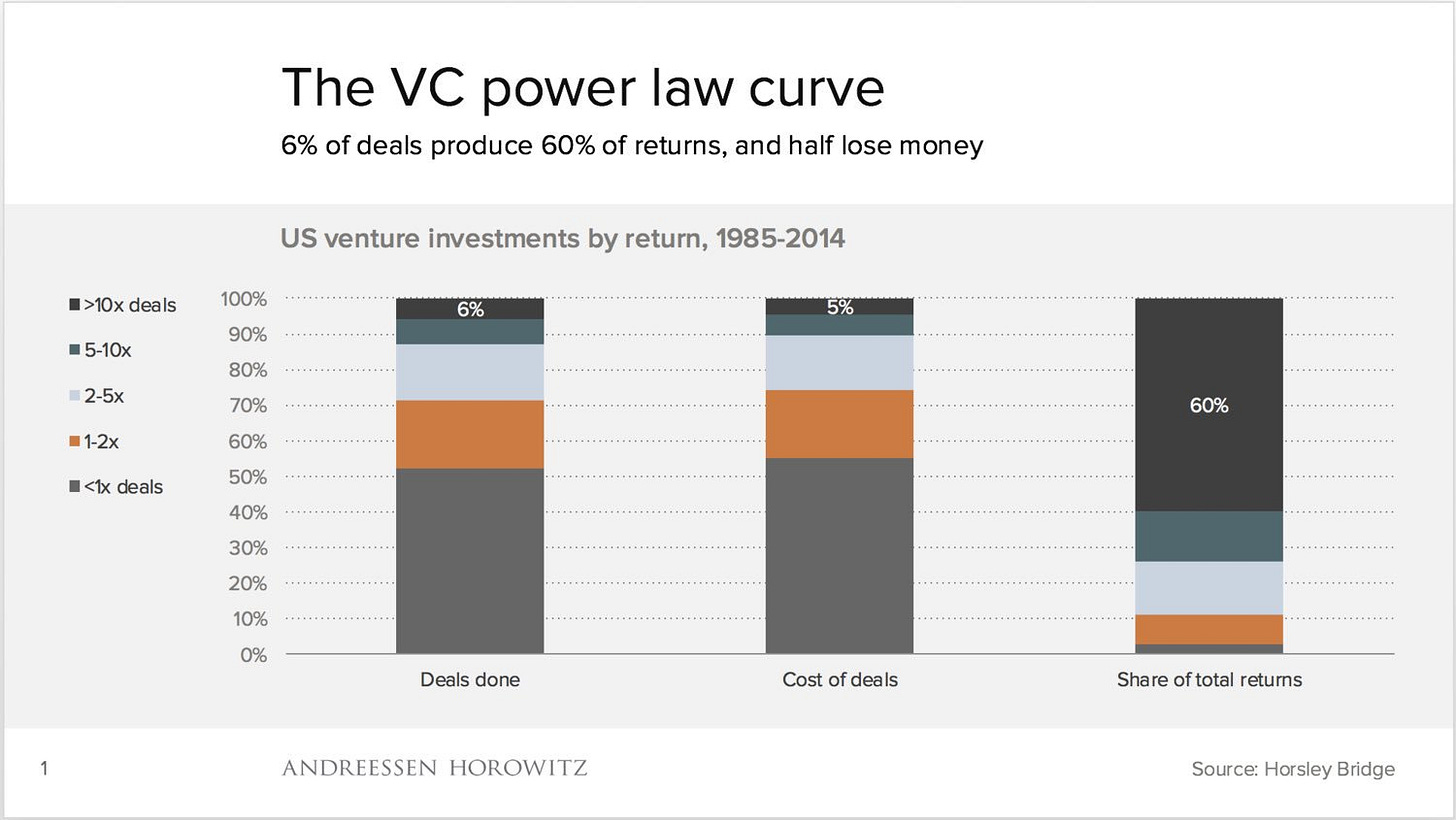
Finally, lets consider the data put out by Angel List. In 2019, they published aggregate returns data of 1,808 investments that have been syndicated through their platform over the past many years. AngelList generated simulations of several 10-company portfolios out of these 1,800-odd investments and came up with the following return distribution (net of fees):
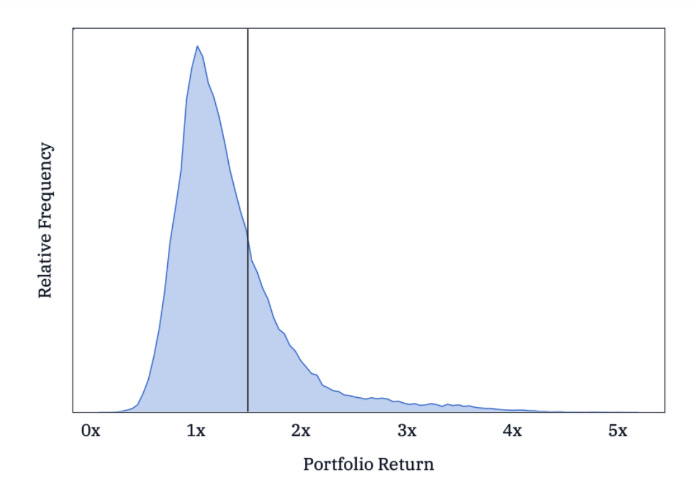
Here’s what Angel List says about this data:
The black vertical line represents the market return, which is what you would get from writing an equal-sized check into all of the 1,808 AngelList investments. All of the probability density to the left of that vertical line represent hypothetical portfolios that underperformed the market return. The most frequently observed outcome from a 10-investment portfolio, the peak of the curve, is slightly positive performance, well under the market return.This is a consequence of the power-law returns of venture capital: the typical manager fails to pick any outsize winners in their 10 chances, whereas the market portfolio is assured of selecting all of the return-driving winners. On the other hand, all of the probability density to the right of that line represents hypothetical portfolios that outperformed the market.
…
A look at VC Funds’ performance
Its quite clear now that VC investments follow a power law. But what about VC firms? Is there a wide dispersion in the quantum of returns seen between firms? Are some firms way more skilled at identifying outliers than others?
In 2015, Quartz published a study on the VC firms which had invested in the most number of ‘unicorns’. Unicorns are startups valued at USD 1 billion and above.For this study, Quartz looked at early-stage investments—seed and series A rounds—in startups that ultimately became billion-dollar companies, using Pitchbook’s US data from 2000 to June 10, 2015.
(Note: An investment definitely does not need to be a unicorn to be an outlier – what constitutes an outlier would also be highly dependent on the fund size. However, for the purpose of this exercise, let’s loosely equate unicorns with outlier investments considering most of the funds cited below are quite large)
Here are the truncated results:
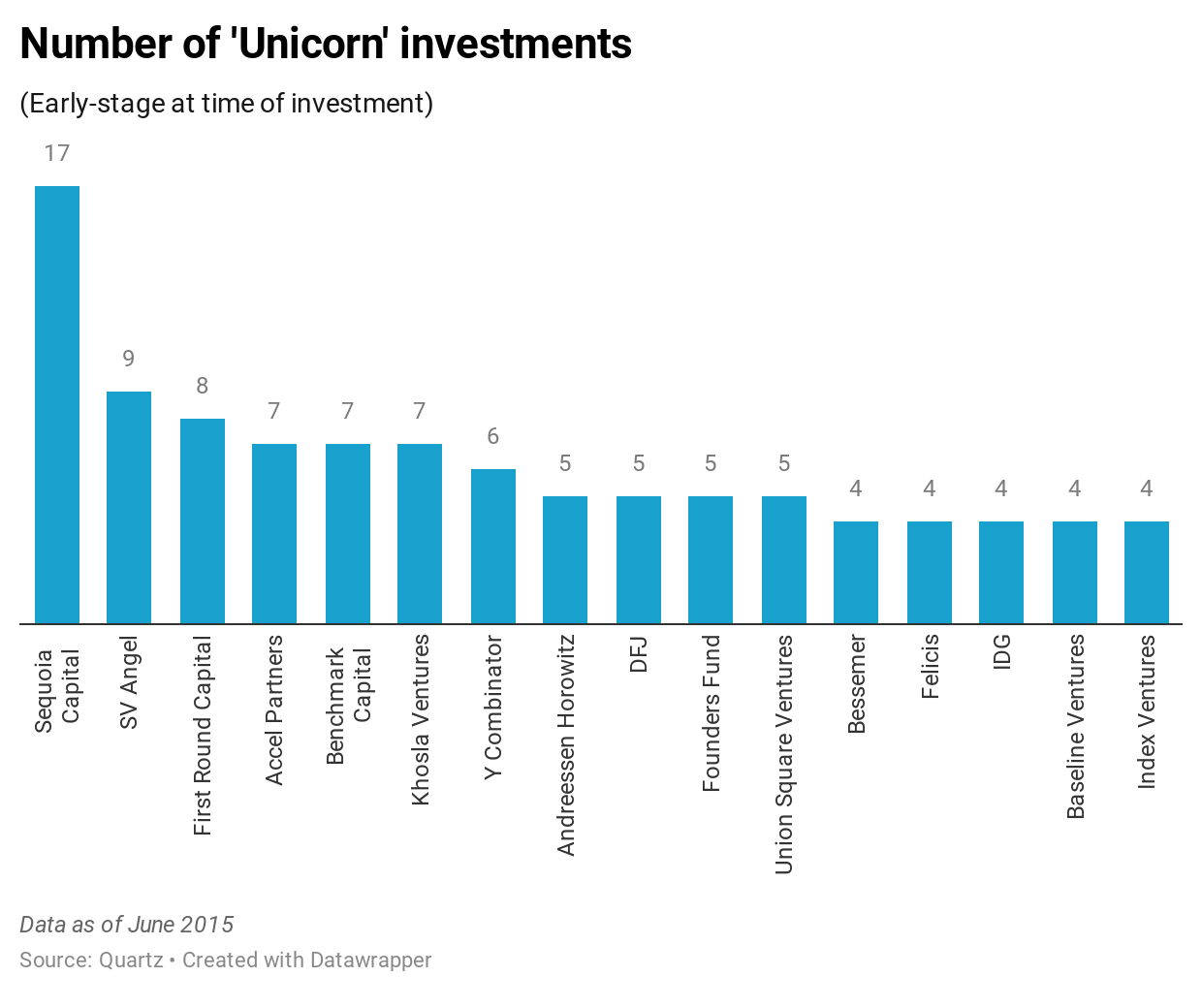
But this gives only the partial picture. The number we should focus on is, what percentage of the investments made by a particular VC firm that turn out to be unicorns.
Here are the results:
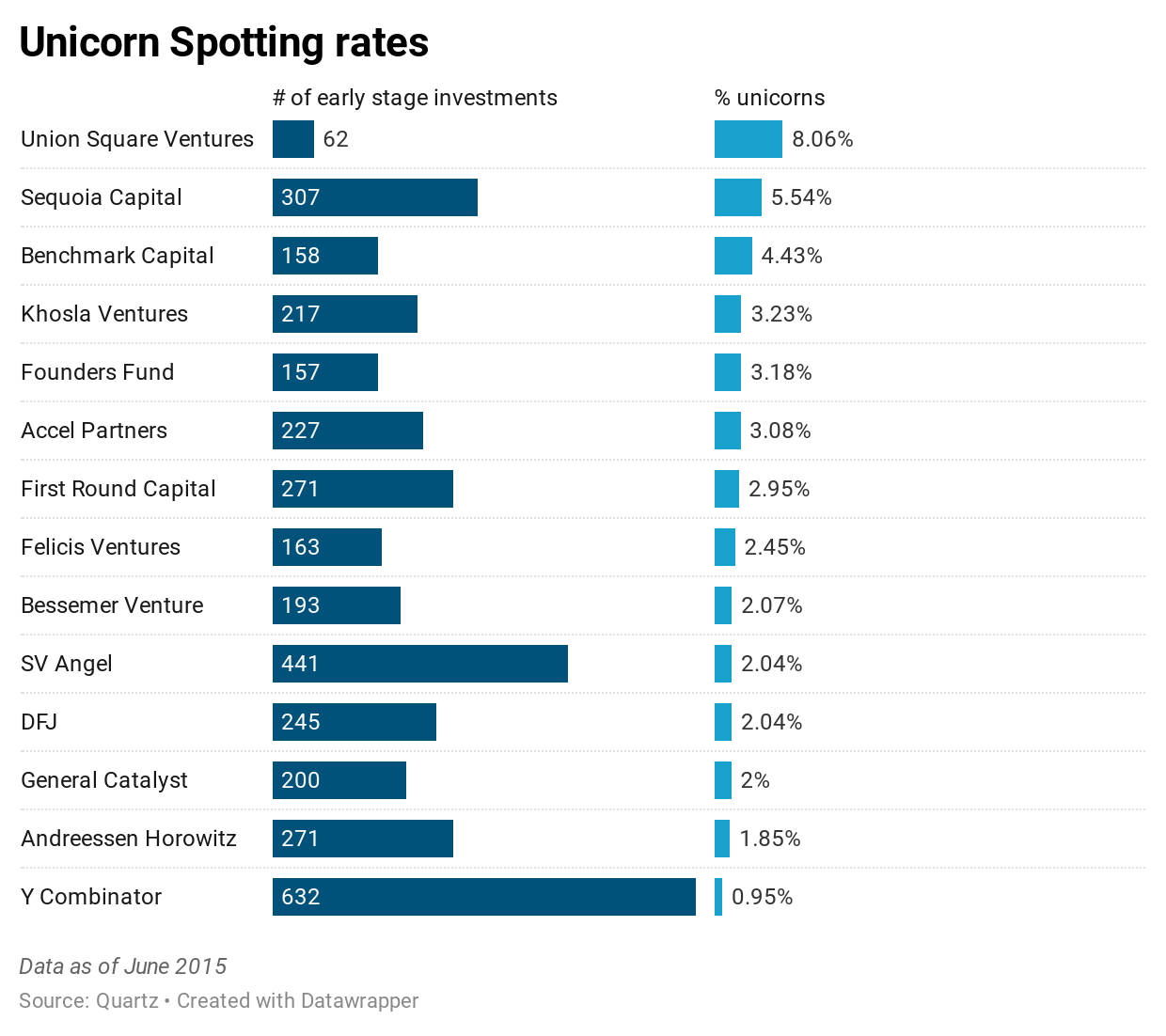
The top 14 VCs have an aggregate of 93 unicorn investments, but this collectively represented only 2.6% of their total number of investments made over the life of these funds. Sequoia Capital, which has invested in the most number of unicorns as per the data, actually saw only 1 in 20 companies reach the USD 1 billion valuation mark.
Almost all the firms that got in early with at least four unicorns tout a rate of 1% to 3%, with a few exceptions. Y Combinator, for example, invested in the most number of early-stage startups (808), deflating its unicorn-spotting rate to 0.7%. The other major exception is Union Square Ventures, which got in early with five startups (Etsy, Twitter, Zynga, MongoDB, Twilio) eventually worth at least $1 billion. It had only invested in 62 early-stage companies—a whopping rate of 8%
…
What does this mean for VC portfolio construction?
Looking at the above data points, it can be reasonably concluded that not only is the venture capital business focused on identifying extreme outliers, but also that even the best funds have way more misses than hits.
In such a scenario, what would an optimum portfolio strategy look like?
Here’s what Peter Thiel, one of the most prominent venture capitalists and partner at Founders’ Fund, says about this –
Given a big power law distribution, you want to be fairly concentrated. …If you invest in 100 companies to try and cover your bases through volume, there’s probably sloppy thinking somewhere. There just aren’t that many businesses that you can have the requisite high degree of conviction about. A better model is to invest in maybe 7 or 8 promising companies from which you think you can get a 10x return…
I was a bit surprised reading this, since my first conclusion after reading about the power law was that VCs would want to build a fairly diversified portfolio rather than a concentrated one.
This is because it is quite clear that it is tough to pick winners – if only 2.5% of the investments are generating the bulk of returns in the industry (as we saw in the Cambridge data above), then what is the possibility of finding + being able to invest in one of them?
Probability of investing in an outlier = Probability of the investment being an outlier * Number of investments made
Since, the first component of the RHS is extremely low (no matter how skilled you are), then logically, it would seem that the best way of increasing odds in your favour would be to focus on increasing the second number which is the number of investments being made.
Let’s see if this argument holds up by doing some simple calculations:
The Cambridge data above puts the odds of success at 2.5% (success being loosely defined here as investing in an outlier). Let’s call this the ‘skill level’ of an ‘average’ fund manager.
Now, lets see how the probabilities of having atleast one outlier in the fund shift at different skill levels and at different number of investments in the fund.
(Note: Probability of having atleast one outlier in the fund = 1 – probability of no outliers in the fund)
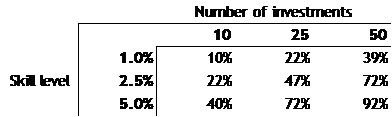
This analysis, while simplistic, shows that an average VC (skill level = 2.5%) can improve his / her odds of investing in an outlier to that of a highly skilled investor just by doubling the number of investments in the fund.
This analysis is simplistic because:
There is perhaps no point in increasing the number of investments unless you have atleast a reasonable level of conviction in the investment
Second, if you actually manage to invest in a home-run, you would not want your fund to be too diversified (say only 2% of the fund is invested in the outlier investment). While the returns may compensate for the ‘over-diversification’, the opportunity cost may be too high.
Third, this analysis completely ignores the ‘value add’ that some funds add to their portfolio companies, either in terms of strategy or operations or hiring or just ‘opening doors.’ To put this in another way, Benchmark and Sequoia make money not only because they invest in ‘good companies’, but also because they help those companies grow.
…
It does seem that having a larger portfolio would, in general, lead to better outcomes for early-stage funds than having a smaller, concentrated portfolio. It is tough to conclude this though, because of so many factors that aren’t (and cannot) be considered in such an analysis.
However, one thing does seem quite clear - If even the top funds manage to hit home runs only 1-3% of the times, competing on skill alone may not be a very wise thing to do in this business. ¯\_(ツ)_/¯
…
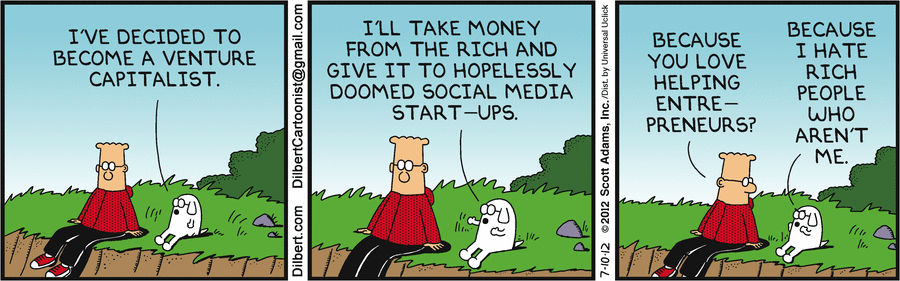
Some interesting reads:
1. How will CRED make money? (Justin Alva)
Unlike most startups which focus on the large addressable market that India offers, CRED has consciously chosen to be a product for the top 1%. Kunal Bahl had famously said this - “If you cannot make revenue with the top 25 million customers, you cannot make money on anything.” Justin does a great job at figuring out what Cred’s business model could look like.
2. The Three Sides of Risk (Morgan Housel)
“There are three distinct sides of risk: The odds you will get hit. The average consequences of getting hit. The tail-end consequences of getting hit…Tail-end events are all that matter. Once you experience it, you’ll never think otherwise.” Don’t miss this article!
References:
Cambridge data: http://cambridgeassociates.com/wp-content/uploads/2015/11/Venture-Capital-Disrupts-Venture-Capital.pdf
Horsley Bridge data: https://a16z.com/2015/06/08/performance-data-and-the-babe-ruth-effect-in-venture-capital/
Angel List data: https://angel.co/blog/what-angellist-data-says-about-power-law-returns-in-venture-capital
Thank you for reading! Please share it with people whom you think might find it interesting :)
And if you wish to receive such posts directly in your inbox, please subscribe. No spam, ever!
You can also follow me on Twitter here.